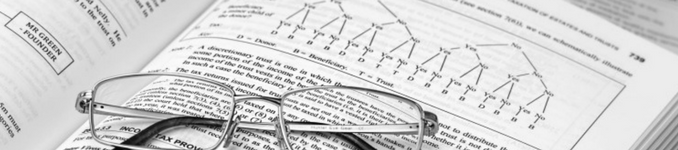
By Susannah Hume, King’s College London and Behavioural Insights Team |
An overarching principle that guides all of BIT’s work is the importance of testing. Although there are many ways to test the effectiveness of a programme, the gold standard is the randomised controlled trial (RCT).
The use of RCT-based field research in public policy domains has been growing over the past few decades, and BIT has been at the centre of this trend; we have conducted more RCTs than the rest of the UK Government in its history, and have published a practitioners’ manual for RCT design: Test, Learn, Adapt.[1]
Testing what works
Say we have the goal of increasing the number of students who are achieving the A’ level grades that would enable them to get accepted to a highly selective university. We have an idea that might help, which could be something like developing a personalised learning plan.
In a general roll-out, we design our learning plan template then develop learning plans with all students who are eligible. We then observe how many students achieve the grades we hope for (the green people in Figure 1).
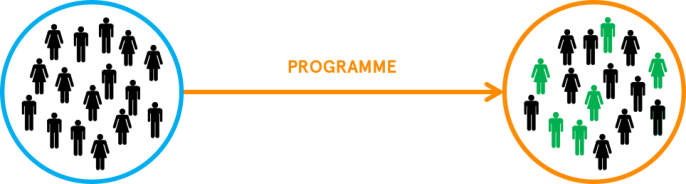
However, in this scenario we don’t know how many of the students who passed would have got those grades anyway, without the learning plan, which means they’ve spent time doing something that hasn’t really made a difference and the time taken to make the plans could have been used for something else that may have been more effective in raising achievement levels.
This is known as a missing counterfactual problem.[2] Basically, because we’ve given the learning plans to all the students, we can never know how those students would have fared if we hadn’t.
Developing a comparison group
To work around this, we need to consider comparison groups, to try and get us as close to the ‘true’ counterfactual as possible. There are a few ways we could do this, including,
- Compare year-on-year (i.e. comparing the cohort that made the plans to the previous year’s cohort).
- Provide learning plans with a sub-group of students or classes (for example, students who apply or are nominated by their teachers), and then compare achievement among that group to the rest of the possibly eligible group).
- Identify a group of students that are demographically similar to those receiving the learning plan; for example, in neighboring sixth forms, or drawn from a national dataset.
However, we cannot rule out the fact that these groups may still be systematically different from one another—for example, one group might have different teaching practices, more engaged or able students, or might for other reasons be more or less likely to benefit from the learning plan.
This, again, means we aren’t comparing like with like, and it’s impossible to tell whether the difference in achievement we observe is because of the learning plan or because of the difference in underlying characteristics of the groups. There are two risks with this:
- The learning plan looks like it works when it has had no effect, because the treated group was more likely than the comparison group to achieve the grades for other reasons–resulting in time and resources being put into something that has no effect, to the detriment of other interventions.
- The learning plan looks like it doesn’t work when it has, in fact, improved things for the treated group, because the comparison group was just more likely to get those grades for other reasons–resulting in an effective intervention being scrapped.
This is known as a problem of selection bias. If people have been selected into the learning plan based on any rule (such as year, class, demography or by application), we cannot be sure that there aren’t other differences between the groups that are driving the results we see. We therefore cannot confidently conclude anything about the effectiveness intervention that we tried to test based on this comparison.
Knowing the causes of things
RCTs present the solution to these challenges. We are no longer selecting our intervention and comparison groups, but rather we’re letting chance decide who receives the intervention.
In simple terms, we take everyone who is eligible for the intervention, and we flip a coin. If it comes up heads, those learners receive the learning plan (in research terms, they are in the ‘intervention’ or ‘treatment’ group), while if it comes up tails, they receive everything else as usual (they are in the ‘control’). We then observe the differences in achievement between the group receiving the learning plan and the group that isn’t, in ‘real time’, to see whether there is more of a grade improvement in the former (see Figure 2).
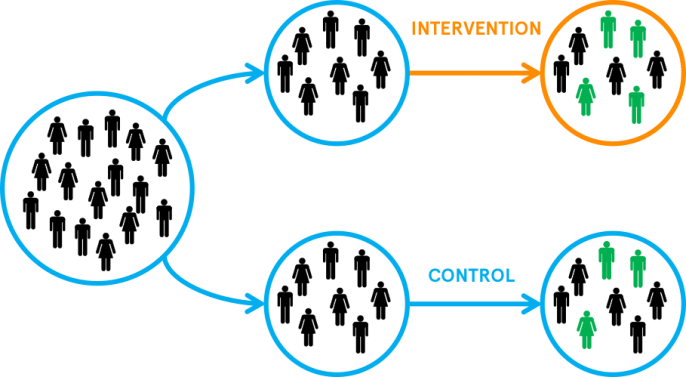
With a sufficiently large sample, we expect that, because of randomisation, the learners in both groups will have the same set of characteristics, including educational background, cognitive and non-cognitive skills, and motivation, and they will experience the same average level of teaching quality, funding support, and external barriers to achievement.
This means that, unlike with other methods of comparison outlined above, we expect that the two groups are identical, on average, before one group receives the learning plan. So when we’re comparing the achievement rates between the groups, we can assume that any difference in achievement is attributable to the intervention.
In other words, the outcomes in the control group are as close as it is possible to get (without crossing into a different universe) to the outcomes we would have observed in the treated group if we hadn’t treated them.
Because of this, RCTs enable us to confidently say what level of increased achievement is caused by personal learning plans. And we can then weigh up, given the cost (in time and funding) of delivering the PLPs, whether they are a good use of learners’ and teachers’ time.
In the second blog post, I talk about the ethics of RCTs, and particularly the commonly-held ethical concern that RCTs require the withholding of an intervention or opportunity from those who would otherwise have received it. I’ll also look at when not to run an RCT.
Click here to join our mailing list.
Follow us on Twitter: @KCLWhatWorks
_________________________________________________________________
[1] https://www.gov.uk/government/uploads/system/uploads/attachment_data/file/62529/TLA-1906126.pdf
[2] The ideal would be to compare each student receiving the learning plan with themselves in an alternate universe where they did not receive the learning plan. This latter (hypothetical) universe would be a situation that is counter to the fact of what happened–hence, the counterfactual.
Leave a Reply